This is a guest post by Lev Tankelevitch, one of my PhD students. He is currently using MEG to explore reward-guided attention at the Oxford Centre for Human Brain activity. This article is also cross-posted at the Brain Metrics.
In 1935,
Hans Berger writes in one of his seminal reports on the
electroencephalogram (EEG), addressing the controversy surrounding the origin of the then unbelievable electrical potentials recorded by him from the human scalp:
 |
Fig. 1. Hans Berger and his early EEG recordings from the 1930s. Adapted from Wiki Commons. |
"I disagree with the statement of the English investigators that the EEG originates exclusively in the occipital lobe. The EEG originates everywhere in the cerebral cortex...In the EEG a fundamental function of the human cerebrum intimately connected with the psychophysical processes becomes visible manifest." (see here for a history of Hans Berger and the EEG)
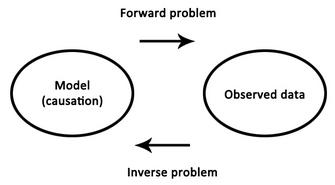 |
Fig. 2. The forward and inverse problems |
Decades later, the correctness of his position is both a blessing and a curse - we now know that the entire brain produces EEG signals, but it has been a struggle to match components of the EEG to their specific sources in the brain, and thus to further our understanding of how exactly the functioning of the brain relates to those psychophysical processes with which Berger was so enthralled. This struggle is best summarised as an
inverse problem, in which one begins with a set of observations (e.g., EEG signals) and has to work backwards to try to calculate what caused them (e.g., neural activity in a specific brain region). A massive obstacle to this approach is the fact that as electrical signals pass from the brain to the scalp they become heavily distorted by the skull. This distortion makes it exceedingly difficult to try to
reconstruct the underlying sources in the brain.
In 1969, the journey to understand the electrical potentials of the brain took an interesting and fruitful detour when David Cohen, a physicist working at MIT, became the first to confidently measure the incredibly tiny magnetic fields produced by the heart's electrical signals (see
here for a talk by David Cohen on the origins of MEG). To do this, he constructed a shielded room, blocking interference from the overwhelming magnetic fields generated by earth itself and by other electrical devices in the vicinity, effectively closing the door on a cacophony of voices to carefully listen to a slight
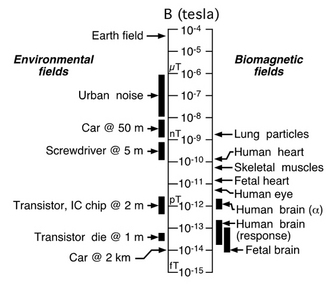 |
Fig. 3. Comparisons of magnetic field strengths on a logarithmic scale. From Vrba (2002). |
whisper. His shielding technique became central to the advent of
magnetoencephalography (MEG), which measures the yet even quieter magnetic fields generated by the brain's electrical activity.
This approach to record the brain's magnetic fields, rather than the electrical potentials themselves, was advanced even further by
James Zimmerman and others working at the Ford Motor Company, where they developed the
SQUID, a superconducting quantum interference device. A SQUID is an extremely sensitive magnetometer, operating on the principles of quantum physics beyond the scope of this article, which is able to detect precisely those very tiny magnetic fields produced by the brain. To appreciate the contributions of magnetic shielding and SQUIDs to magnetoencephalography, consider that the earth's magnetic field, the one acting on your compass needle, is at least 200 million times the strength of the fields generated by your brain trying to read that very same
compass.
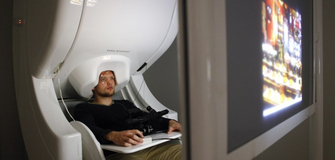 |
Fig. 4. A participant being scanned inside a MEG scanner. From OHBA. |
A MEG scanner is a large machine allowing participants to sit upright. As its centrepiece, it contains a helmet populated with many hidden SQUIDs cooled at all times by liquid helium. Typical scanners contain about 300 sensors covering the entirety of the scalp. These sensors include
magnetometers, which measure magnetic fields directly, and
gradiometers, which are pairs of magnetometers placed at a small distance from each other, measuring the difference in magnetic field between their two locations (hence "gradient" in the name). This difference measure subtracts out large and distant sources of magnetic noise (such as earth's magnetic field), while remaining sensitive to local sources of magnetic fields (such as those emanating from the brain). Due to their positioning, magnetometers and gradiometers also provide complementary information about the direction of magnetic fields.
Given that these magnetic fields occur simultaneously with electrical activity, MEG is afforded the same millisecond resolution as EEG, allowing one to examine neural activity at its natural temporal resolution. This is in contrast to
functional magnetic resonance imaging, fMRI, which, using magnetic fields as a tool rather than a target of measurement, actually measures changes in blood oxygenation which occur on the order of seconds, making it impossible to effective pinpoint the time of neural activity (see
here). Another advantage over fMRI is the fact that electromagnetic signals are more directly related to the underlying neural activity than the
haemodynamic response, which may differ across
brain regions, clinical populations, or with respect to
drug effects, thereby complicating interpretations of observed effects. Unlike the electrical potentials measured in EEG, however, the magnetic fields measured in MEG pass from the brain through the skull in a relatively undisturbed manner, substantially simplifying the inverse problem. In these ways, for a non-invasive technique, MEG best combines high temporal resolution and improves source localisation within the human brain.
What exactly do those tiny magnetic fields reflect about brain activity? When a neuron receives communication from a neighbour, an excitatory or inhibitory postsynaptic potential (
EPSP or
IPSP) is generated in the neuron's dendrites, causing that local dendritic membrane to become
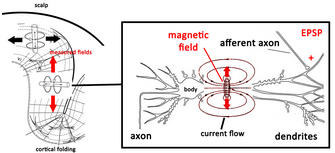 |
Fig. 5. The source of recorded magnetic fields in MEG. Adapted from Hansen et al. (2010) |
transiently depolarised relative to the body of the neuron. This difference in potential generates a current flow both inside and outside the neuron, which creates a magnetic field. One such event, however, is still insufficient in generating a magnetic field large enough to be detected even by the mightiest of SQUIDs, so it is thought that the fields measured in MEG are the result of at least 50,000 neurons simultaneously experiencing EPSPs or IPSPs within a certain region. Unfortunately, current technology and analysis methods are limited to detecting magnetic fields generated along the cortex, the bit of the brain closest to the scalp. Fields generated in deeper cortical and subcortical areas rapidly dissipate as they travel much longer distances through the brain. To complicate things further, we have to remember that magnetic fields obey Ampère's
right-hand rule which states that if a current flows in the direction of the thumb in a "thumbs-up" gesture of the right hand, the generated magnetic field will flow perpendicularly to the thumb, in the direction of the fingers. This means that only neurons oriented tangentially along the skull surface generate magnetic fields which radiate outwards in the direction of the skull to be measured at the surface. Fortunately, mother nature has cut scientists some slack here, as the pervasive folding pattern (
gyrification) of the brain's cortex provides us with plenty of neurons arranged in the direction useful for MEG measurement. The cortex alone is enough to keep scientists busy, and findings from fMRI and direct electrophysiological recordings from non-human animals provide complementary information about the world underneath the cortex, and how it may all fit together.
At the end of a long and arduous MEG scanning session, one is left with about 300 individual time series, typically recorded at 1000 Hz, reflecting tiny changes in magnetic fields driven by neural activity presumably occuring in response to some cognitive task. Although the shielded room blocks out magnetic interference from other electrical devices (and all equipment inside the room works through
optical fibres), there is still massive interference from the subject's heart and any other muscle activity around the head. For this reason, participants are typically instructed to limit eye movements and blinking and any remaining artefactual noise in the data (i.e., anything not thought to be brain activity) is taken out at the analysis stage using techniques like
independent component analysis.
 |
Fig. 6. Raw MEG data (left), and event-related fields in sensor space and source space (right). Adapted from Schoenfeld et al. (2014). |
Analysis of MEG data can be done in
sensor space, in which one simply looks at how the signals at individual sensors change during different parts of a cognitive task. This provides a rough estimate of the activation patterns along the cortex. The perk of MEG, however, is the ability to project data recorded in the 300 sensors to
source space, and effectively estimate where in the brain these signals may originate. Although this is certainly more feasible in MEG than EEG, the inverse problem is actual a fundamental issue to both types of extracranial recordings (we don't have this problem when measuring directly from the brain during
intracranial recording). One way to narrow down which possible activation regions in the brain could underlie the observed magnetic fields is to establish certain assumptions about
what we expect brain activity to look like in general, and how that activity is translated into the signal measured at the scalp. Such assumptions are more reasonable in MEG than EEG due to the higher fidelity of magnetic fields as they pass from the brain to scalp.
 |
Fig. 7. Neural activation is smooth, forming clusters of active neurons. Adapted from Wiki Commons. |
For example, neural activation in the brain is assumed to be
smooth. Imagine all the active neurons in a brain at a single point in time as stars in the sky: smooth activation would mean that the stars would form little clusters, rather than appear completely randomly all over the sky. Indeed, this feature of brain activation is what allows us to detect any magnetic fields using MEG in the first place! Remember that only many neurons within a local region which happen to be simultaneously active generate fields strong enough to be detected at the scalp.
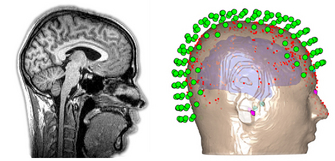 |
Fig. 8. MRI structural image of the head and brain (left), and sensor, head, and brain model (right). Adapted from Wiki Commons and OHBA. |
Another assumption is that the fate of the travelling magnetic fields depends on the physical size, shape, and organisation of the brain and scalp. To this end, MEG data across all 300 sensors are registered to an MRI scan of each participant's head and a 3D mapping of their scalp (obtained by literally marking hundreds of points along each participant's scalp using a digital pen), which together provide a high spatial resolution description of the anatomy of the entire head, brain included. These assumptions, among others, are used to mathematically estimate where in the brain the measured magnetic fields may have originated at each point in time.
 |
Fig. 9. Alpha, beta, and gamma oscillations. Adapted from Wiki Commons. |
There are two general approaches when analyzing MEG data. Analysis of event-related fields looks at how the timing or the size of the magnetic
fields changes with respect to an event of interest during a cognitive task (e.g., the appearance of an image). The idea is that although there is a lot of noise in the measurement, if one averages many trials together the noise will cancel out, while the effect of interest, which always occurs in relation to a precisely timed event in the cognitive task, will remain. This follows in the tradition of EEG analysis, in which these evoked responses are called
event-related potentials. Alternatively, one can use
Fourier transformations to break the data down into frequency components, also known as waves, rhythms, or
oscillations, and measure changes in their phase or amplitude in response to cognitive events. This follows in the tradition established by Berger himself, who discovered and named
alpha and
beta waves. Neural oscillations have recently received a lot of attention as they are suggested to be involved in
synchronizing the activity of populations of neurons, and have been associated with a number of cognitive functions such as
attentional control and
movement preparation, as in the case of alpha and beta oscillations, respectively.
Other resources:
For a slightly more in-depth description of MEG, see
here.
For a more in-depth description of MEG acquisition, see this
video.
And for the kids, see this excellent article at
Frontiers for Young Minds
References
Baillet, S., Mosher, J.C., & Leahy, R.M. (2001).
Electromagnetic brain mapping. IEEE Signal Processing Magazine..
Fernando H, Lopes da Silva. MEG: an introduction to methods. eds: Hansen, Kringelback & Salmelin. USA: OUP, 2010:1-23, figure 1.3 from p6.
La Vaque, T. J. (1999). The History of EEG Hans Berger: Psychophysiologist. A Historical Vignette.
Journal of Neurotherapy.
Proudfoot, M., Woolrich, M.W., Nobre, A.C., & Turner, M. (2014). Magnetoencephalography.
Pract Neurol, 0, 1-8.
Schoenfeld, M.A., Hopf, J-M., Merkel, C. Heinz, H-J., & Hillyard, S.A. (2014). Object-based attention involves the sequential activation of feature-specific cortical modules.
Nature Neuroscience, 17(4).
Vrba, J. (2002). Magnetencephalography: the art of finding a needle in a haystack.
Physica C, 368, 1-9.
The data figures are from papers cited above. All other figures are from Wiki Commons.